Steps to AIOps maturity: Improve MTTR with AI
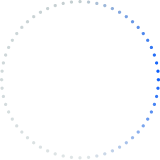
Many organizations face increased costs from excess noise, manual workflows, and long outage times. These inefficiencies negatively impact budget, service uptime, and, ultimately, customer satisfaction.
With effective use of AI, you can give operators the most relevant, full-context incident data, providing a greater understanding of an incident within seconds. According to research from Enterprise Management Associates, respondents report that alerts from mature AI programs are 75% to 100% actionable, supporting proactive incident responses and reducing outage frequency.
However, implementing AI isn’t as easy as turning on a light switch. To see actual results, you need to take the time to build a foundation of structured, accessible data. In our previous posts on reducing alert noise and establishing actionable incidents, we discuss the first phases of reaching AIOps maturity. In this third phase, you start to reap the benefits of your work in the first stages.
Implementing AI into your workflows
The work in the initial phases ensures that you have high-quality alert data, allowing you to uncover patterns and provide visibility across your infrastructure to support more accurate generative AI results. Teams can then use GenAI to summarize insights, identify impacts, and understand root causes to enhance overall incident management.
Three core ways you can use operations-centric AI tools to improve mean time to resolution MTTR include:
- Automated Incident Analysis: Built on normalized and correlated incident data, GenAI populates high-quality, relevant summaries in natural language for additional incident insights. Automated Incident Analysis helps your teams quickly understand causality and impact, dramatically shortening resolution time.
- Root-Cause Analysis: AI automatically identifies the changes that may have caused IT incidents and provides real-time suggestions about how to resolve them. Along with tracking changes proactively, AI monitors and adjusts the Root-Cause Change configuration to improve and customize match suggestions.
- Similar Incidents: Need a reality check that you’re making the right next step? AI populates historical analysis of relevant past incidents so you can cross-reference impact, priority, and steps to resolution for new incidents. Using Similar Incidents, your teams can confirm that incident tags are up-to-date and enabled for entity, problem, impact, and topology to ensure accurate similarity scoring.
Driving measurable results with AI
When implemented correctly, AI can streamline workflows and automate processes. Each organization has unique priorities and goals. Some of the more significant success metrics you can track with AI are:
- Reducing costs
- Improving operator efficiency
- Maximizing service reliability
- Shortening MTTR
On a more granular level, you can continue to utilize BigPanda Unified Analytics dashboards to spot trends in change data as well as MTTx and efficiency improvements. Specific dashboards such as Change Analysis use your data to spot recurring issues to fix within your infrastructure.
Customer example: Chipotle accelerates root-cause analysis
The Covid business environment dramatically increased Chipotle’s online orders, forcing its IT team to seek more efficient ways to enhance incident triage and address incidents in real time.
“BigPanda funnels our alert data, identifies incidents in real-time, and automatically builds out full context tickets so the appropriate team is alerted for incident triage, cutting our MTTR in half.”
Joe Connelly
Chipotle Mexican Grill
To expedite and simplify root-cause identification, it’s essential to understand where manual efforts fall short and identify areas for strategic process enhancements. Joe Connelly, director of monitoring, observability, and service reliability at Chipotle Mexican Grill, had his team spend time in phases 1 and 2 of the AIOps journey, cleaning up data to power high-quality, actionable alerts.
Once the team ensured data cleanliness, Chipotle began automating processes. Connelly emphasized the significance of utilizing tools such as generative AI to reduce unnecessary IT noise and accelerate root-cause analysis. The Chipotle ITOps team progressed through the AIOps maturity phases step-by-step. They now have full-context data and automated root-cause investigation, triage, and incident resolution.
Setting forth with AIOps
The journey to AIOps maturity and success can be challenging, but you don’t need to do it alone. BigPanda helps its customers succeed, no matter what your data quality looks like today. BigPanda’s best-in-class event-management platform reduces noise and seamlessly integrates GenAI so you can automate workflows and see real benefits to operational efficiency.
Next steps
- See firsthand how other organizations are navigating Phase 1. Our recent customer webinar covers best practices for event normalization and alert enrichment.
- Download “A practical guide to AIOps maturity” for an overview of all phases and more resources.
“Observability is a journey. BigPanda AIOps is a key part of this journey for us. As we scale and grow the business, it’s integral for us to bring in automation and integration with other tools and technologies. My recommendation is don’t wait to start your AIOps journey once you are overwhelmed with alerts. Start early to get a single pane of glass to understand which monitoring tools you really need.”
Sanjay Chandra
Vice President of Information Technology, Lucid Motors