Why event correlation, and how is AIOps involved?
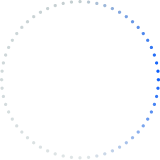
Event correlation and AIOps go hand-in-hand. Event correlation is the process of identifying patterns in data that may indicate a problem or opportunity. As defined by Gartner, AIOps—short for artificial intelligence for IT operations—combines big data and machine learning to automate IT operations processes, including event correlation, anomaly detection and causality determination.1 In this blog post, we’ll explore the importance of event correlation and how artificial intelligence (AI) is involved. We’ll also dispel some common misconceptions about event correlation in AIOps.
What is the importance of event correlation?
Enterprises today are dealing with an ever-growing volume, variety and velocity of data. This data comes from a wide variety of sources, including event data from applications, servers, storage systems, networking equipment and more. It can be in the form of performance data, log files, application telemetry, system alerts and other events. Humans may struggle to find patterns or trends that could be suggestive of issues or possibilities amid such a large quantity of data.
This is where event correlation comes in. Event correlation can help to identify patterns in data that you would not be able to see otherwise. It can also help to automate the analysis of this data so you can get the information you need, when you need it.
Event correlation can be used for a variety of purposes, including:
- To identify problems: If you have a lot of data, it can be difficult to identify when something is going wrong. Event correlation can help you to identify patterns that may indicate a problem. For example, if you see a spike in error messages from a particular application, this may be indicative of a problem.
- To diagnose problems: Once you have identified a problem, event correlation can help you to diagnose the root cause. For example, if you see that error messages are coming from a particular application, you can use event correlation to find out what is causing the errors.
- To prevent problems: Event correlation can also be used to prevent problems from occurring in the first place. If you see that a particular application is prone to errors, you can use event correlation to find out what is causing the errors and then take steps to prevent them from happening.
- To find opportunities: Event correlation can also be used to identify patterns that may indicate opportunities. If you see that a particular application is being used more often than others, this may be an indication that it is popular and you may want to promote it.
What are the benefits of event correlation?
There are many benefits to using event correlation, including:
- Improved situational awareness: Event correlation can help you to identify patterns that you would not be able to see otherwise—enabling you to be more aware of what is going on in your environment and make better decisions.
- Early detection of problems: Event correlation can help you to identify problems early, before they cause major issues. This can help you to avoid outages and other disruptions.
- Reduced mean time to repair (MTTR): Event correlation can help you to diagnose problems quickly and accurately—helping you to reduce the time it takes to repair them.
- Improved incident response times: Event correlation can help you to identify problems quickly and determine the root cause—helping you respond to incidents more quickly and resolve them more effectively.
- Better capacity planning: Event correlation can help you to identify patterns in data that can help you to plan for future capacity needs.
How is AI involved in event correlation?
AI is playing an increasingly important role in optimizing core enterprise operations, from marketing and customer service to supply chain management and security. Because of innovation in machine learning (ML) and deep learning technologies, which are capable of automatically identifying patterns in data, AI is being used more and more for event correlation. But first, let’s go over machine learning and deep learning.
Machine learning is a type of AI that allows computers to learn from data without being explicitly programmed. ML algorithms are designed to automatically find patterns in data and improve their performance over time.
Deep learning is a type of machine learning that uses artificial neural networks to learn from data. Deep learning algorithms are able to automatically learn complex patterns in data and improve their performance over time.
The aim of using AI for event correlation is to automatically identify patterns in data that may indicate a problem. By using machine learning and deep learning algorithms, businesses can quickly find problems and take steps to prevent them.
There are a number of benefits to using AI for event correlation, including:
- Automated pattern recognition: Identify patterns in data—helping to identify problems and opportunities that you would not be able to see otherwise.
- Automatic root cause analysis: Automatically diagnose the root cause of problems, ultimately helping to resolve problems more quickly and effectively.
DevOps teams and enterprises can use AI for event correlation to improve the efficiency and effectiveness of their operations.
Event correlation in AIOps
Here’s how event correlation integrates with AIOps.
AIOps was created in response to the challenges that businesses face in managing today’s complex IT environments. The goal of AIOps in event correlation is to accelerate the identification and resolution of IT issues. By using AIOps, businesses can quickly find and fix problems before they cause major disruptions.
Key components of AIOps platforms include:
- Open box machine learning: Correlates alerts, changes and topology data, to reduce noise and detect evolving incidents as they happen, before they escalate.
- Open integration hub: Ingests monitoring, change and topology data for full-stack visibility and alert enrichment with out-of-the-box connectors.
- Event enrichment engine: Enriches alerts with topological and operational information collected from all technology domains, allowing AI and machine learning to detect incidents.
- Automatic incident triage: Uses custom tags to add business context to incidents, enabling teams to rapidly triage incidents and automate next steps.
- Root cause changes: Uses AI/ML to identify recent changes that may be causing an incident, and provides the reasoning behind every decision in simple language.
- Real-time topology mesh: Ingests topology data from all sources of topology in the environment to provide an up-to-date, visual, full-stack topology model.
- Incident 360 console: Creates shared awareness for ITOps, NOC, DevOps and SRE teams by providing a 360-degree overview of each incident detected.
- Unified analytics: Includes ready-to-use ITOps reports and dashboards and provides end-to-end insights into health trends and ITOps key performance indicators (KPIs).
- Enterprise cloud platform: SaaS-native platform provides built-in scaling, seamless updates, high availability and SOC-2 compliance with lightning-fast provisioning.
As AIOps matures, event correlation will become more important for predicting trends and uncovering underlying causes that people may overlook. AI-driven event correlation technologies will be able to seamlessly connect into incident management systems and advise decision makers on what to do and how to accomplish it.
Enterprises can harness the power of event correlation through AIOps to manage today’s complex IT environments. By automating the identification and resolution of IT issues, AIOps enables enterprises to improve service levels, avoid outages, reduce operational costs and ultimately deliver better customer experiences.