Accelerate root-cause analysis with AIOps
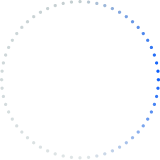
The digital landscape is evolving constantly — as is its complexity. Organizations need more efficient and effective ways to sort through high volumes of IT noise to identify the root cause of incidents. In a recent webinar with BigPanda CIO Jason Walker and Waste Management Principal Architect Udo Strick, Joe Connelly — director of monitoring, observability, and service reliability at Chipotle Mexican Grill — shared his perspective on:
- IT challenges faced in a pre-AIOps era
- The importance of clean, high-quality data for investigation and root-cause analysis
- How generative AI will impact standard practices of incident management in the future
Pre-AIOps constraints of manual processes in root-cause analysis
Before delving into current AIOps strategies, the speakers discussed the challenges they faced in a pre-AIOps IT environment. For Connelly and his team at Chipotle, the lack of visibility into real-time data delayed incident knowledge and triage.
“BigPanda funnels our alert data, identifies incidents in real-time, and automatically builds out full context tickets so the appropriate team is alerted for incident triage, cutting our MTTR in half.”
Joe Connelly
Chipotle Mexican Grill
“Five years ago, the Chipotle mobile app was up and coming, and we started to gain traction in the digital space. When Covid hit in 2020, we hit a new peak of 50% of sales coming from the mobile app,” explained Connelly. “As mobile use grew in popularity, so did the growth of alerts and complexities happening on the back end.”
At the time, the system refreshed alert reports with updated sales data hourly. “If average sales were abnormally low for that time of day, we inferred an incident was taking place that prevented mobile ordering,” he said. “That was our only cue to troubleshoot as quickly as possible to get the mobile app back online.”
Recognizing the increasing importance of the mobile app in satisfying hungry customers, Chipotle sought more efficient ways to enhance incident triage. The goal was to identify and address incidents closer to real-time, which meant moving away from the hour-by-hour waiting period for incident confirmation and resolution.
“We had gone as far as human efforts could manage,” shared Connelly. “This led us to explore AIOps, and ultimately BigPanda.”
Clean data fuels high-quality, actionable alerts
Another critical objective for the Chipotle team was to secure a consistent cadence of data that had been scrubbed of redundant, substandard information automatically. High-quality, full-context data ensures the team always has a clear view of the IT environment. Respective ITOps teams can identify incident root cause quickly and align the right person to the right task for speed resolution.
Meeting this objective required gaining immediate insight into the current status of the IT environment, says Connelly. “BigPanda funnels our alert data, identifies incidents in real-time, and automatically builds out full context tickets so the appropriate team is alerted for incident triage, cutting our MTTR in half.”
By implementing full-context operations, teams can enhance information sharing to smooth communication and eliminate fragmented knowledge across departments. Filtering data at its origin and throughout its progression ensures superior data quality and facilitates swift, effective incident triage.
Workflow automation accelerates root-cause analysis
The success of automating any process hinges on data quality. Connelly emphasized the significance of reducing unnecessary IT noise to accelerate root-cause analysis, cautioning against automating multiple processes until you’ve assured data cleanliness. Ensuring clean data is vital to making confident decisions — automation with inconsistent data can undermine results.
“I tend to call it garbage in, garbage out. If you put garbage — or poor, irrelevant data — into a product, any product, you’re not going to get high-quality output from it,” says Connelly. “When we filter out this low-quality alert noise, we don’t lose record of that data. But it does get flushed out, making room for the root cause of an issue to be clearly identified and presented to the right team for resolution.”
Continual enhancement with AI
Connelly’s strategic vision with AIOps is to look at tools such as generative AI, believing they will soon become the standard practice of automating root-cause investigation, triage, and incident resolution.
“I was originally skeptical of AI because some AI tools just felt like hype,” he said. “Today, though, generative AI is very impressive, and if you look at realized potential, we have a long runway. Generative AI is something that we cannot ignore, so we are actively looking at the next steps.”
Automate IT incident management for faster resolution
To expedite and simplify root-cause identification, it is essential to first understand where manual efforts fall short and identify areas for strategic process enhancements. BigPanda Alert Intelligence streamlines millions of events into a few actionable alerts, no matter where they originate. ITOps teams get a centralized view to confirm incident root cause in real-time and determine the necessary actions for rapid triage. To go even deeper with your root cause analysis efforts, BigPanda combines your high-quality, enriched IT alert data with the latest GenAI innovations to automatically and reliably reveal critical incident analysis, incident impact, and probable root cause in natural language.
Next steps
- Watch the full on-demand webinar to learn more about root-cause analysis from Connelly and Strick.
- To learn more about getting to root cause in real-time for faster triage, read “How automated root-cause analysis can help reduce MTTR.”
- Explore how BigPanda Generative AI enriches IT alert data to autonomously deliver vital incident analysis, impact assessment, and likely root causes in plain language.