Black Box Machine Learning Ate My Homework
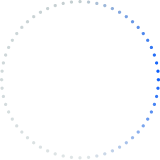
This is the first in a series of blog posts on Open Box Machine Learning.
If you’re part of a large enterprise, you’re probably in the throes of digital transformation.
If you’re in IT, you’re supporting your business by rolling out new services and apps weekly (or even daily). Meanwhile, your users expect 24×7 availability and performance.
So your IT operations team is having to sift through ever-increasing data pouring out of myriad specialized and fragmented monitoring tools, hybrid clouds, legacy systems and virtual infrastructure. To make some sense of it all, your front line operators try to manually correlate and isolate issues before your users – and your business – are impacted… all the while hoping you don’t miss something critical.
You realize that this solution is neither scalable nor cost-effective, not to mention the risks it creates to your business from headline-grabbing outages and unhappy, vocal users.
At the recently concluded Gartner IOSS Summit in Orlando, FL, Gartner recognized that effective incident management is one of the critical issues that Infrastructure and Operations (I&O) leaders inside large enterprises struggle with, every day. Gartner’s recommendation to these dynamic enterprises was to leverage intelligent automation and increasingly more autonomous systems to tame the Big Data problem in IT.
(Read our show report)
That’s why we created BigPanda Autonomous Digital Operations Platform: to reduce operational costs, increase service availability and reduce the risk of digital transformation initiatives. Autonomous Digitial Operations is part of AIOps, in case you are wondering, but a much more precisely defined application of advanced machine learning. BigPanda correlates, automates and streamlines incident management in the face of ever-increasing complexity, while its results delight your users.
As we built the BigPanda Platform, knowing the mission-critical role IT Operations teams play inside large enterprises, we chose to be guided by three overarching principles:
- Respect for our customers
- Empathy for their IT Operations teams
- Quick time to value, not marketing hype
These principles and their ethos permeates all aspects of who we are and what we do here at BigPanda. Nowhere is this more evident than in our approach to data science – which we call Open Box Machine Learning, the engine that powers the BigPanda Platform.
What is Open Box Machine Learning?
Open Box Machine Learning is BigPanda’s unique and highly pragmatic implementation of autonomous intelligence.
It applies a variety of machine learning techniques in highly unique ways to process IT incidents in real-time across contextual dimensions. It continually suggests new, ever-more-efficient automation logic. And it lets IT Ops teams incorporate their hard-won, real world knowledge into the logic it creates.
In a recent article by PwC’s Artificial Innovation Accelerator practice, the authors argue that exposing how AI does what it does – opening the “black box” as it were – is necessary to build greater trust in the technology. For example, how does AI know what action to complete or decision to make? How can companies prevent it from making a mistake? PwC advises that AI experts “must take steps to help people understand how AI learns. People also should understand exactly what is behind AI’s reasoning and decision-making once it has learned how to perform its intended function.”
They assert 3 keys to open up machine learning magic to closer scrutiny, as this figure illustrates…
This is exactly what sets BigPanda’s Open Box product philosophy apart. Our users can examine our automation logic in plain English, edit this automation logic as needed, and then preview it before deploying to production. This is quite different from the approach taken by alternative AIOps vendors that employ a closed, black box machine learning approach whose inner workings are opaque and obscured from IT Ops users.
Using our Platform, enterprise IT can reduce up to 95 percent of its operational noise, improve SLA compliance by more than 50 percent, and meaningfully improve on operational KPIs such as MTTD, MTTA and MTTR. Don’t take our word for it, our customers happily share their own success.
Black Box Machine Learning Ate My Homework
The choice between Open Box or Black Box machine learning is a choice that each organization must make for itself. Ultimately it boils down to a difference in operational philosophy. Our customers are large, complex global enterprises. They rightly want to take advantage of IT automation technologies, with machine learning initiatives ranking among the top CIO priorities for 2018. But they want to do it on their own terms, in a way that is fully transparent and controllable, so they can safely entrust critical aspects of their IT operations to BigPanda.
No IT leader responsible for optimizing digital operations, ..we’re willing to bet, would prefer to entrust a single aspect of their IT operations to a black box machine learning solution that dabbles in “unknown unknowns” (to borrow a turn of phrase from Don Rumsfeld). AI that can produce a different result every single time it runs does little to fulfill PwC’s guidance of transparency, explainability and provability.
Why?
When you have a headline-grabbing outage on your hands, it’s hard to explain to your CIO that you placed your trust in black box AIOps that missed a critical incident. Data scientists call this kind of result “non-deterministic”, meaning that the underlying logic just decides on its own how to respond each time it processes a data set of IT alerts. Your results may vary.
Why?
When you have a prolonged outage on your hands, it often requires a bridge call from hell. In IT we define that as a 4+ hour troubleshooting call with 150 operators, domain experts and engineers on the line. That’s exactly what one of our customers had to contend with, before switching to BigPanda… and that’s all too typical! Incident resolution goes a lot quicker and smoother when black box AIOps doesn’t send you down a rabbit hole with 53 percent confidence in root cause. There goes IT’s credibility with your business stakeholders.
Why?
When you have an unexplainable outage on your hands, then Level 1 operators – the human first responders to IT incidents – start to feel uneasy because, well, the black box machine learning works in mysterious ways!
“Black box machine learning made me do it” is just another version of “the dog ate my homework”. That excuse never flew with your teachers back in school, and it won’t work today with your IT managers, customers and business execs either.
If at First You Don’t Succeed, Test, Test Again
Isaac Sacolick, an influential consultant to CIOs on digital transformation, advised in a recent column for CIO Magazine that, when evaluating winning technology platforms, it’s wise to ask: “Does the platform enable experimentation and testing? … While vendors provide different tools to extend their platforms, one key differentiator is to evaluate how easy it is to test changes before they are pushed into production environments.”
We assert that, here in 2018, if machine learning’s efficacy can’t be demonstrated to an IT Ops prospect in a proof of concept implementation (what we call a POV) as a condition of purchase – then it’s a black box filled with marketing hype and empty promises. What’s worse, if it takes 12 months to implement an AIOps solution effectively, how “intelligent” is that? These vendors are doing a disservice to IT Operations when mission-critical transformation initiatives are at stake.
We’re humbled that some of the largest companies in the world – including one of the largest airlines in the world, one of the largest semiconductor manufacturers in the world, and one of the largest media conglomerates in the world – trust us and our Open Box Machine Learning approach to simplify their IT operations. We work hard every single day to earn their trust.
Getting machine learning right is hard (that’s why it’s practitioners are called data scientists!) Making machine learning easy to use, easy to test, and easy to customize is extremely hard. Making it dependable and trustworthy even more so. It has taken us here at BigPanda years of rigorous R&D and product engineering to get it right. Every time we hear that our approach helped our customers trust that the answers coming from machine learning were correct – or that they avoided another “bridge call from hell” – we’re more convinced that the majority will want to automate their incident management from an Open Box.
LEARN MORE:
- AI & Machine Learning Black Boxes: The Need for Transparency and Accountability (Knowledge Discovery Nuggets)
- 8 Ways Machine Learning Is Improving Companies’ Work Processes (Harvard Business Review)
- How Artificial Intelligence will Transform IT Operations and DevOps (Ronald Van Loon)