AI-driven contextual mastery for incident response
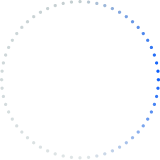
Context is fundamental to well-run tech operations, which require an understanding of systems, services, architectures, and teams to interpret the real-time data streaming in from observability and change systems. The delivery of context is crucial for effective operations performance. And it’s a universally important skill set for tech Ops teams to master.
Gaining this awareness and understanding often requires lengthy, inconsistent hands-on experience observing systems and people react under stress, rather than formal training and onboarding. Teams struggle to update their understanding as systems and services undergo constant updates and migrations. It’s an unsolved problem.
Modern services are large, complex, and vital to every enterprise. Ops teams face an unending stream of uninformative, disconnected alerts every day. They spend precious minutes or hours researching each one, escalating and reassigning, collectively struggling to gather enough context to act with confidence and precision to keep those services running. The organizational consequences are both acute and chronic.
In an acute example, an Ops team receives numerous high CPU temperature alerts affecting different applications. The team searches for internal load-related causes, only to discover — hours into the investigation — that a heat wave caused regional brownouts and a subsequent HVAC failure at the data center. The remediation is unexpectedly a failover to other infrastructure, which causes an acute impact (downtime). The team can take action only once everyone has enough context to understand that’s the right choice.
In a more chronic example, a new operations engineer escalates a recurring critical APM event to a senior engineer without knowing it’s a known false positive that triggers multiple times every day, leading to mutual frustration and inefficiency.
Not all context is created equal
The tools and processes that most organizations use today aren’t up to the task of delivering full context. Their tools create gaps in incident response and frustrations for the teams involved and lead to operational churn and poor service availability.
The variety of relevant contextual information — and the systems and people that hold that information — are what make it so difficult. Some of the most critical types of context include:
- Source Context: The first questions that operations engineers ask focus on building context. Where do they start? The source. They look at the entity named in the event, perhaps a host, app, or container, and then at the condition that triggered the event, which provides the initial, limited context relatively quickly.
- Situational Context: This is about what’s happening in the rest of the environment that may be related and connecting the dots. What other events, incidents, updates, patches, or maintenance may be related to this one? What load is on the service? Are internal shared services healthy? How are third-party services looking? This level of situational awareness is hard to achieve momentarily in enterprises, let alone consistently.
- Historical Context: Involves sifting through multiple systems of record to recognize meaningful patterns amidst vast data volume, emphasizing the necessity of identifying relevant information from routine records for effective incident response.
- Topological Context: It’s not just understanding the systems and components but the interconnections between them. It’s akin to deciphering an intricate map. And it’s vital to diagnose issues, plan changes, and anticipate ripple effects so that you can maintain and update your systems without causing additional issues.
- Remediation Context: Successful remediation typically relies on what’s in runbooks and in the brains of experienced responders. It’s challenging, if not impossible, to combine those in the fast fury of an active incident. It highlights a paradox at the heart of ITOps: The most critical knowledge is often the hardest to formalize and disseminate.
- Human Context: This extends beyond on-call schedules to service ownership, domain expertise, historical incident activity, and accountability across teams. It’s knowing who owns what, who knows what, and how to navigate the social and professional landscapes of the organization to act quickly and confidently.
In the realm of IT operations and incident response, integrating these diverse contexts is vital to maintain operational integrity over time and through constant change. While professionals navigate source, situational, historical, topological, remediation, and human contexts daily, their outdated tools, organizational silos, knowledge gaps, and varied operator experience hinder their efficacy.
An answer to the challenge
The introduction of generative AI offers transformative capabilities to revolutionize operational effectiveness by bringing all that context together in near-zero time, with absolute consistency. This innovation promises a future where operations teams are empowered by instant AI-driven insights, complementing and strengthening — rather than replacing — human expertise.
Imagine a world where Ops teams don’t have to spend part or all of each day retrieving the right event and incident context from disparate knowledge management systems, volumes of historical tickets and post-mortems, or siloed domain experts.
In pursuit of achieving full context, the BigPanda Innovation team has developed the BigPanda AI-powered copilot, currently in beta. Codenamed “Biggy,” the copilot not only uses machine-generated and historical data, but is the first to leverage all sources of human-generated, institutional knowledge for AI-powered incident response.
This broad spectrum of knowledge aggregated by BigPanda, known as the Unified Data Fabric, allows Biggy to deliver automatic, dynamic, and actionable insights to ITOps and ITSM teams as they investigate and respond to live incidents.
Next-level intelligence
The BigPanda AIOps copilot is more than just a chat interface into event data or a new way to search knowledge systems. Its capabilities reach much further. For instance, the copilot:
- Provides a flexible, interactive collaboration partner for all operations functions, including historical operations analysis and real-time diagnostics on your services
- Aggregates relevant data intelligently from documentation, chat histories, knowledge articles, and traditional operations systems
- Integrates into the point of use for operations teams, allowing you to get important AI-powered insights right in your chat tools, ticketing systems, and the BigPanda console
The copilot streamlines communication, collaboration, and decision-making in ITOps. Beyond faster response times, it enables higher service availability and operational efficiency. And it increases the capability of the operations function within the enterprise.
With Biggy, we seamlessly blend human insights with AI precision. With it, enterprise IT teams will be better equipped to handle every event that comes their way — quickly and competently. Not only will this drive faster incident investigations and resolutions to keep services up and running, but it will also redefine operational effectiveness and evolve to meet the demands of modern technology landscapes.
The BigPanda AIOps copilot is currently available to select customers as part of an early access program.
If you want to explore how AI-powered incident response can revolutionize your IT operations management, contact your BigPanda account team to be a beta partner on the new AIOps copilot. Stay tuned for more updates on how this new product will deliver full context ops to enterprise IT teams, enabling you to reach unprecedented incident response and service availability goals.