The future of pragmatic AI
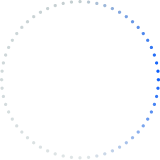
It’s no secret that every ITOps leader can face an ever-increasing amount of alerts. Since the dawn of digital, alerts have served an important purpose. Sometimes all those alerts can become overwhelming noise, and sorting out what is and is not a priority can become challenging. The good news is that AI and machine learning (ML) are adept at processing large data sets in real-time, looking for patterns, and being able to aid in decision-making. With AI on your side, that tsunami of alerts you’re dealing with now in ITOps can be turned into a rippling stream.
How AI is utilized today
AI and ML are perfectly suited to ITOps and could aid teams everywhere in controlling their alerts and outages. The great thing is that ITOps teams already have many of the tools and data they need for AI to be successful. Many observability tools and vendors have large data sets and linear regressions that outline trends and past events.
Along with data points, AI can mine historical tickets and other data for predictive analytics—like when a ticket is likely to appear and who it should be assigned to. It can also utilize past data to expertly know who is on call and who to page instead of paging (and potentially waking) an entire DevOps team.
The BigPanda approach to AI
BigPanda’s AIOps (artificial intelligence for ITOps) platform has been applied in multiple ways and can drive real value without relying on expensive data science. Before incidents even occur, BigPanda’s AIOps platform can intelligently detect them in real time before they escalate into outages. If there’s an incident whose cause is undetermined, BigPanda can help determine the root cause. At the end of the day, AI and machine learning is a design philosophy. Some of the largest organizations in the world use BigPanda’s AIOps Event Correlation and Automation platform, and it processes 30 billion events each year.
The future of pragmatic AI
There’s a huge opportunity in utilizing pragmatic AI, and BigPanda plans to continue to help organizations in all stages of AI adoption. There will be three main areas of focus: automated feature configuration to further accelerate deployments using the ITOps data cloud and AI; automated feature optimization to improve performance over time with recommendations based on data; and real-time predictions.
Pragmatic AI has real value for ITOps teams, and AI and ML can assist at any stage of the incident lifecycle. In the meantime, BigPanda will continue to focus on automation and optimization for real-time predictions.