How observability and AIOps work better together
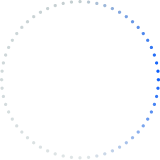
If you’re juggling complex, cloud-based, containerized systems and aiming to meet high customer expectations, your old monitoring processes probably don’t cut it anymore. Increasing infrastructure complexity means you need to instrument more, log more, and monitor more. That leads to even more complexity. The answer is better observability, right?
Yes and no. Observability and monitoring are critical, but they are only part of what you need for service awareness and availability. 91% of organizations report challenges when deploying observability solutions and observability tools alone cannot automate tasks, reduce alert noise, prioritize alerts, or automate incident resolution responses.
That’s where AIOps platforms come in. According to ESG, 55% of organizations deploy an AIOps solution as part of their observability practice. That’s not surprising, given that AIOps enhances observability solutions by automating tasks, streamlining alerts, and quickly resolving incidents.
Using AIOps with your observability tools can sift through data to detect unusual patterns, ensuring IT teams focus on top-priority issues. AIOps also provides early alerts for potential problems and suggests proactive solutions. In essence, AIOps elevates the efficiency of observability, monitoring, and event management.
Discover the differences between these tools and learn how AIOps helps to eliminate noise from your observability data sources for more actionable incidents, improved productivity, and increased automation.
How can you use observability and AIOps together?
Using observability with AIOps can provide deep insights into system behaviors through AI-driven analysis. It transcends traditional observability tool limitations by automating complex data interpretations and identifying anomalies. Consequently, AIOps enhances system understanding, enabling more proactive and informed decision-making.
Why do observability tools need AIOps?
Metrics, logs, and trace data are very noisy and observability tools are not designed to identify and separate actionable alerts from unnecessary noise. That’s where AIOps comes in, to automatically remove observability noise and identify important, actionable alerts.
In addition, AIOps analytics help improve your observability strategy and identify improvements that make your observability tool more actionable. Using AIOps with observability tools also can identify the changes that cause incidents, a major source of outages in today’s modern dynamic and hybrid IT stacks. This assists your teams with incident remediation and resolution.
BigPanda partner Cribl says “Observability is defined as a concept, a goal, and direction that will help your organization to gain the most insight from the data you can collect. It helps companies diagnose performance issues and resolve issues before they become more significant.”
AIOps can significantly enhance observability tools in several ways, the top six being:
- Root cause analysis: AIOps analyzes complex relationships within an IT environment and helps identify the root causes of performance or availability problems. This accelerates troubleshooting and MTTR (Mean Time To Resolve) and downtime.
- Predictive analysis: AIOps can forecast potential issues before they impact system performance. By analyzing historical data and patterns, it can provide insights and recommendations to prevent future problems.
- Data contextualization: AIOps platforms can enrich IT data with data from other sources and the events coming from observability tools. By combining observability information with other sources like CMDB and Topology data, observability becomes actionable.
- Automation: AIOps can assist you in automating routine operational tasks, such as scaling resources and restarting services, based on insights from observability tools. This reduces manual intervention and enhances efficiency.
- Intelligent Alerts: AIOps help in fine-tuning alerting mechanisms, reducing the noise generated by your observability tools to ensure that operators receive meaningful incidents that require immediate action.
- Support IT tool consolidation: AIOps streamlines IT operations by consolidating multiple observability tools and automating complex tasks, promoting a more cohesive and efficient operational framework. Additionally, AIOps applies advanced analytics to observability data, offering clear insights into observability and monitoring tools themselves.
Using AIOps with observability tools is complementary and provides advanced analytics, automation, and intelligence to help your organizations proactively manage and improve their IT operations. This combination leads to better system performance, reduced downtime, and increased operational efficiency from your NOC and SRE team.
View the Combat Tool Sprawl webinar.
Use BigPanda AIOps with observability for better efficiency
BigPanda is not an observability vendor, but the average BigPanda customer is collecting data from around 20 observability and monitoring tools! Companies using BigPanda greatly enhance observability by aggregating and enhancing data from their many observability tools and measuring the quality of data those tools deliver.
As you onboard observability tools, BigPanda can help by providing clear metrics for comparing tool quality, actionability, and efficiency. Because BigPanda is technology agnostic, separate from your observability vendors, you get clear metrics you can trust to prove and improve the value of your observability investments.
BigPanda is designed to ingest data streams from most existing monitoring and observability tools with minimal configuration.
- BigPanda Open Integration Hub: One of the many ways BigPanda AIOps help your observability tool is with the BigPanda Open Integration Hub, which aggregates alerts in the detection phase from all observability and monitoring tools regardless of the vendors.
- Automatically correlate alerts into incidents: BigPanda correlates enriched alerts into high-level incidents using unique open-box machine learning technology, so you can detect incidents in real-time. Take it from BigPanda customer FreeWheel, who was able to reduce alert noise by over 90%.
According to Cribl, “Observability Tools: To choose the best observability tool, go with one that is flexible, easy to use, and reduces additional costs.”
Observability key factors | BigPanda solution |
Flexibility | BigPanda is agnostic, supports your existing tooling, and is can help with measuring new and existing observability and monitoring tools |
Ease to use | BigPanda has a central place to view and analyze actionable data from all your observability and monitoring tools |
Reduced cost | BigPanda eliminates unnecessary noise and toil, freeing up your team to address important tasks and meet service level goals |
AIOps enhances observability to drive system awareness
AIOps, using algorithms and machine learning, supports observability by processing system data, identifying irregularities, and facilitating automated responses. This level of observability offers IT teams an improved view of system performance and potential issues. Therefore, by using AIOps and observability tools, businesses gain better awareness, helping them address challenges and enhance operational processes.
The solution is BigPanda, an Operational Intelligence and Automation platform, that correlates events into insight-rich and actionable incidents. See the power of AIOps for yourself in our personalized demo. For better awareness, efficiency, and automation of your complex, containerized, multi-cloud infrastructure, combine BigPanda with your observability to drive better quality, value, and, ultimately, business-improving service quality.
1 Enterprise Strategy Group. (2023). Distributed Cloud Series: Observability and Demystifying AIOps
2 Enterprise Strategy Group. (2023). Distributed Cloud Series: Observability and Demystifying AIOps